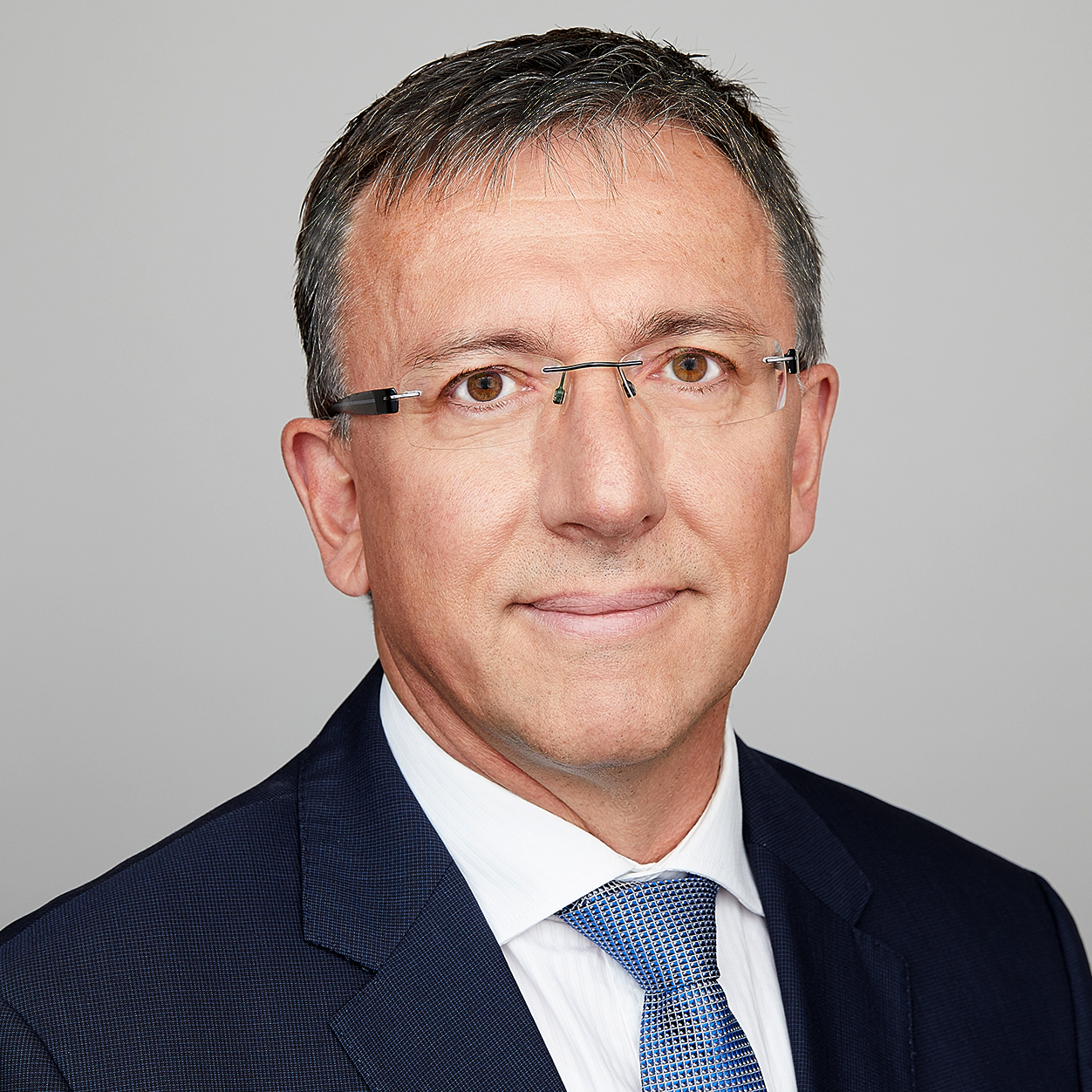
Peter Augat
Professor of Biomechanics at Paracelsus Medical University, Salzburg, Austria and
Director Institute for Biomechanics, Berufsgenossenschaftliche Unfallklinik
Murnau, Germany
Lecture: Towards a clinical pathway for computational planning of operative stabilization of fractured bones
Peter Augat is a Professor of Biomechanics at Paracelsus Medical University in Salzburg, Austria and the Director of the Institute of Biomechanics at Berufsgenossenschaftliche Unfallklinik Murnau, Germany. He received his MSc in physics from the University of Ulm in 1990 and obtained his Ph.D. in biology in 1995. His previous positions include Head of the Osteoporosis and Fracture Healing Research Group at the Institute of Orthopaedic Research at the University of Ulm and Assistant Adjunct Professor in the Department of Radiology at the University of California, San Francisco. Peter is Past President of the International Society for Fracture Repair (ISFR), Past President of the German Society of Biomechanics (DGfB), and Member of several societies in the area of musculoskeletal research including ESB, ORS and IBMS. He is on the editorial board of several orthopaedic journals including Biomedical Engineering, AOTS, JOT, EJTS and also reviewer for most major musculoskeletal journals. Peter’s research is focused on biomechanics of fracture repair and on the clinical translation of orthopaedic research. He published more than 200 scientific articles and supervised more than 80 doctoral theses.
.
Towards a clinical pathway for computational planning of operative stabilization of fractured bones
Operative treatment of broken bones involves their mechanical stabilization using a metallic implant. The primary goal of operative stabilization (i.e. osteosynthesis) is to provide the bone with sufficient mechanical stability to allow functional rehabilitation of the patient. The art of operative fracture care involves choosing the adequate implant and applying the appropriate techniques to reach the goal of sufficient mechanical stabilization. However, an accurate estimation of the implant’s load-bearing capacity is often challenging because of complex loading scenarios and difficult to predict the interaction between bone and metallic implant. Modern computer technology, including finite element analysis, has shown to be capable of accurately calculating the mechanical performance of a fractured bone stabilized with a metallic implant. Yet, clinical reality has not yet seen the use of this technique. This presentation will demonstrate how the mechanical performance of fracture stabilization can be computed and how the existing barriers to translate this technology into the clinical application can be overcome.
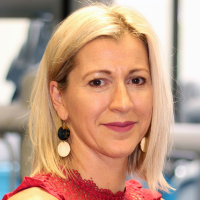
Merryn Tawhai
Professor of Bioengineering, Auckland Bioengineering Institute
University of Auckland
Lecture: Linking imaging-based lung structure to patient respiratory system function using biophysically-based models
Professor Merryn Tawhai is the Director of New Zealand’s national Medical Technologies Centre of Research Excellence and Deputy Director of the Auckland Bioengineering Institute at the University of Auckland. She has led efforts to develop a quantitative description of lung anatomy and accompanying multi-scale models that bring together cell function, tissue mechanics, and transport and exchange processes in anatomically-detailed models of the lung. Her research links patient-specific lung structure to function for simulation of ventilation distribution, perfusion distribution, and gas exchange; as well as simulation of a range of pulmonary function tests. Professor Tawhai is a Fellow of the Royal Society of New Zealand Te Apārangi (RSNZ), the American Institute for Medical and Biological Engineering, the International Academy of Medical and Biological Engineering. She was awarded the 2016 MacDiarmid Medal by the RSNZ for the potential impact of her research on human health.
Linking imaging-based lung structure to patient respiratory system function using biophysically-based models
There are currently very few methods for quantitative assessment of the lung to assist with planning surgical or other interventions. Current tools focus on image analysis, usually based on densitometry or texture analysis. No tools are currently available for patient-specific prediction of respiratory system function post-treatment. We are developing an imaging-, statistical-, and biophysically-based lung model to predict patient-specific response to various interventions and treatments. This digital lung spans from the nasal and oral airways to the deepest smallest parts of the lung, and connects to sophisticated models of the pulmonary circulation, respiratory and other gas exchange, and interacts with models of respiratory control. The model incorporates imaging and lung function data from healthy subjects aged 20-90 years of age to appropriately represent structure-function relationships over the full adult lifespan. The ultimate goal is to provide a comprehensive tool that can be used to predictively test interventional approaches and therapies (both well in advance and at the bedside), provide mechanistic insight into imaging-based biomarkers, and to develop and optimise new and current treatments to the individual patient.
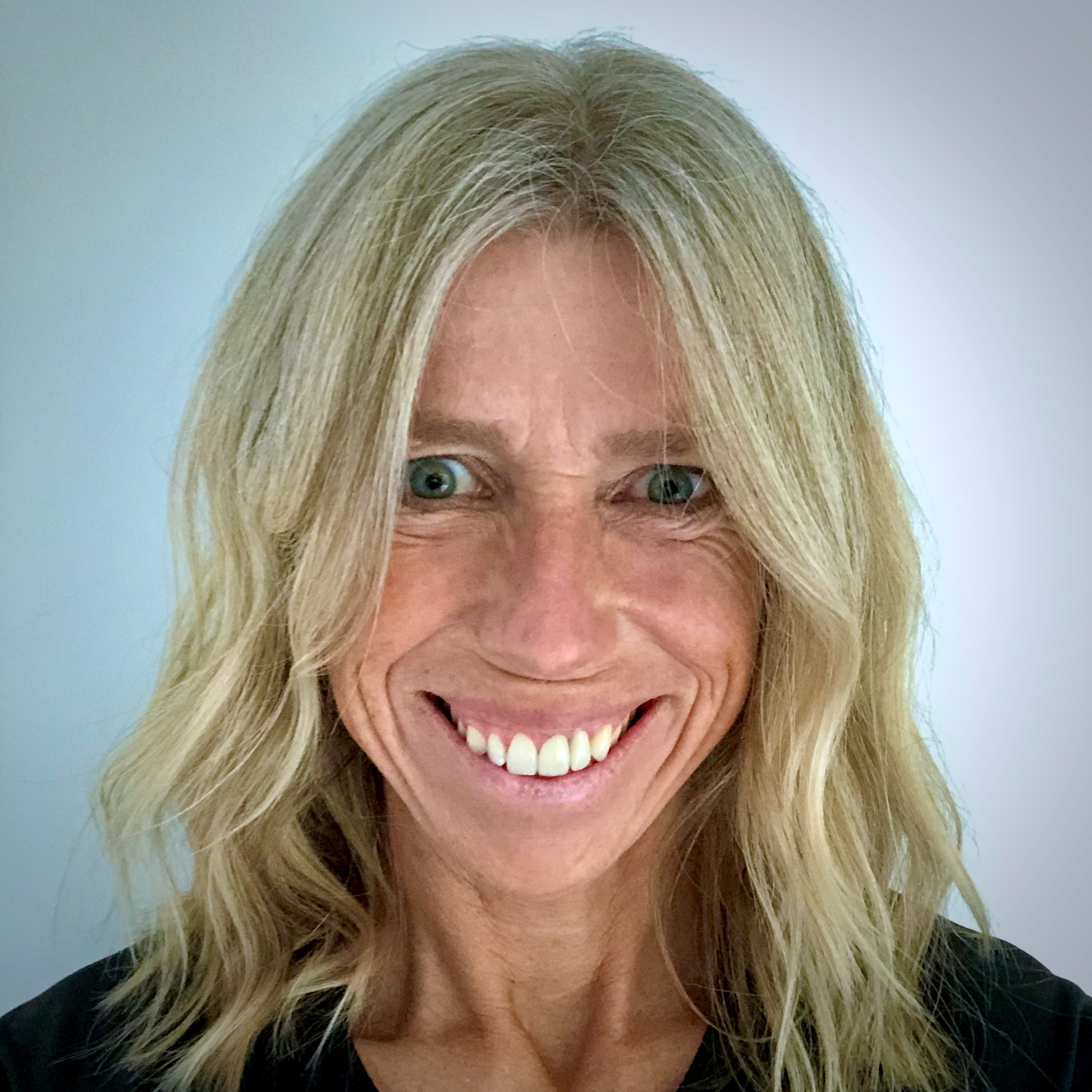
Ellen Kuhl
Robert Bosch Chair of Mechanical Engineering, Professor of Mechanical Engineering and, by courtesy, of Bioengineering Stanford University
Lecture: Data-driven modelling of neurodegeneration
Ellen Kuhl is the Robert Bosch Chair of Mechanical Engineering at Stanford University. She received her PhD from the University of Stuttgart and her Habilitation from the University of Kaiserslautern. Her area of expertise is Living Matter Physics, the design of theoretical and computational models to predict the behavior of living structures. She has published more than 250 peer-reviewed journal articles. Ellen is the current Chair of the US National Committee on Biomechanics and a Member-Elect of the World Council of Biomechanics. She is a Fellow of the American Society of Mechanical Engineers and of the American Institute for Mechanical and Biological Engineering. She received the NSF Career Award, the Humboldt Research Award, and the ASME Belytschko Award. Ellen is an All American triathlete, a multiple Boston, Chicago, and New York marathon runner, and a Kona Ironman World Championship finisher.
Data-driven modelling of neurodegeneration
Neurodegeneration will undoubtedly become a major challenge in medicine and public health because of demographic changes worldwide. More than 45 million people are living with dementia today and this number is expected to triple by 2050. Recent studies have reinforced the hypothesis that the prion paradigm, the templated growth and spreading of misfolded proteins, could help explain the progression of a variety of neurodegenerative disorders. However, our current understanding of prion-like growth and spreading is rather empirical and the precise propagation dynamics of misfolded protein within the living brain remain poorly understood. Here we show that a physics-based reaction-diffusion model can explain the growth and spreading of misfolded protein in a variety of neurodegenerative disorders. We integrate the classical Fisher-Kolmogorov equation for population dynamics into a brain network model, which we represent through a connectivity-weighted Laplacian graph created from 418 human brain images. To test the hypothesis that misfolded proteins propagate preferably along neuronal connections, we follow 46 subjects for three years and compare their positron emission tomography scans against brain network models of intracellular and extracellular spreading. For each subject, we identify a personalized set of model parameters that characterizes the individual progression of misfolded tau protein. We show that intracellular spreading along neuronal connections explains the propagation dynamics of Alzheimer’s disease better than extracellular spreading. Our results suggest that misfolded proteins in neurodegenerative disorders grow and spread according to a universal law that follows the basic physical principles of nonlinear reaction and anisotropic diffusion. A more quantitative understanding of the timeline of neurodegeneration could help detect non-clinical symptoms at an earlier stage and make informed predictions about the timeline of neurodegeneration on an individual personalized basis.
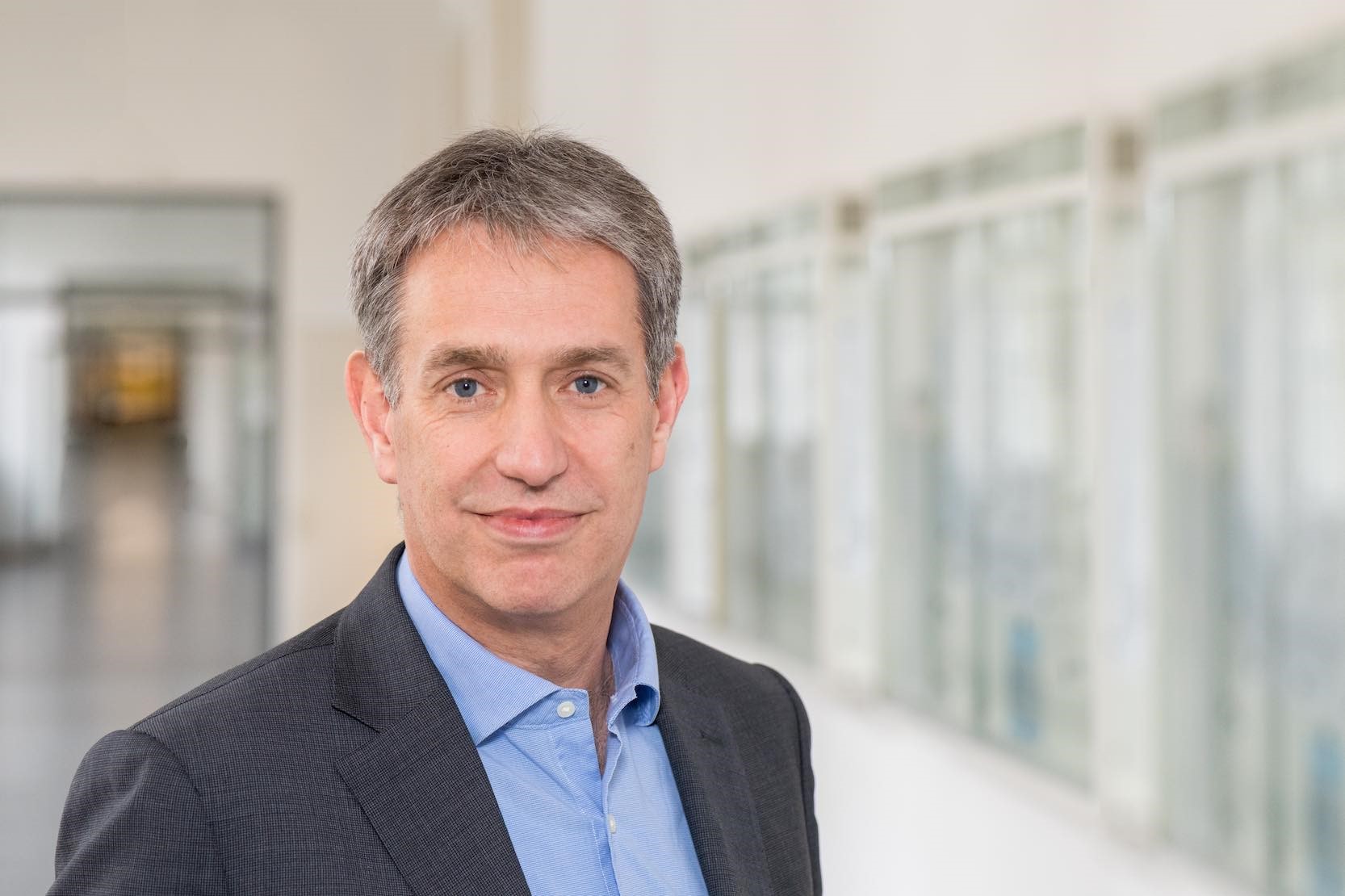
Daniel Rueckert
Professor of Visual Information Processing, Department of Computing, Imperial College London, UK
Lecture: How AI is changing the future of radiology
Daniel Rueckert is Alexander von Humboldt Professor for AI in Medicine and Healthcare at the Technical University of Munich. He is also Professor of Visual Information Processing in the Department of Computing at Imperial College London. From 2016 to 2020 he served as Head of the Department of Computing at Imperial College. He gained a MSc from Technical University Berlin in 1993, a PhD from Imperial College in 1997, followed by a post-doc at King’s College London. In 1999 he joined Imperial College as a Lecturer, becoming Senior Lecturer in 2003 and full Professor in 2005. At Imperial, he has founded the Biomedical Image Analysis group consisting of four academics, 15 post-docs and 20 PhD students. He has published more than 500 journal and conference articles as well as graduated over 50 PhD students. In his early research career he has worked on the development of non-rigid registration algorithms for the compensation of tissue motion and deformation. The developed registration techniques have been successfully used for the non-rigid registration of various anatomical structures, including in the breast, liver, heart and brain and are currently commercialized by IXICO, an Imperial College spin-out company. Professor Rueckert is an associate editor of IEEE Transactions on Medical Imaging, a member of the editorial board of Medical Image Analysis, Image & Vision Computing, MICCAI/Elsevier Book Series, and a referee for a number of international medical imaging journals and conferences. He has served as a member of organising and programme committees at numerous conferences, e.g. he has been General Co-chair of MMBIA 2006 and FIMH 2013 as well as Programme Co-Chair of MICCAI 2009, ISBI 2012 and WBIR 2012. In 2014, he has been elected as a Fellow of the MICCAI society and in 2015 he was elected as a Fellow of the Royal Academy of Engineering and as fellow of the IEEE. More recently has been elected as Fellow of the Academy of Medical Sciences (2019).
How AI is changing the future of radiology
This talk will explain how AI is changing the future of radiology. In particular, I will examine the impact of deep learning on the entire medical imaging pipeline: First, I will introduce a framework for reconstructing MR images from undersampled data using a deep cascade of convolutional neural networks to accelerate the data acquisition process. We show that such a method can outperforms state-of-the-art compressed sensing approaches, both in terms of image quality and reconstruction speed. In addition, we will present how deep learning can be used for image enhancement (e.g. super-resolution) and image quantification (e.g. image segmentation). Finally, we will show examples of how deep learning can be used for diagnosis and prognosis of diseases.